Better object detection by filtering extra unlabeled data with RUPL
Better object detection by filtering extra unlabeled data with RUPL
Semi-Supervised Object Detection with Object-wise Contrastive Learning and Regression Uncertainty
arXiv paper abstract https://arxiv.org/abs/2212.02747
arXiv PDF paper https://arxiv.org/pdf/2212.02747.pdf
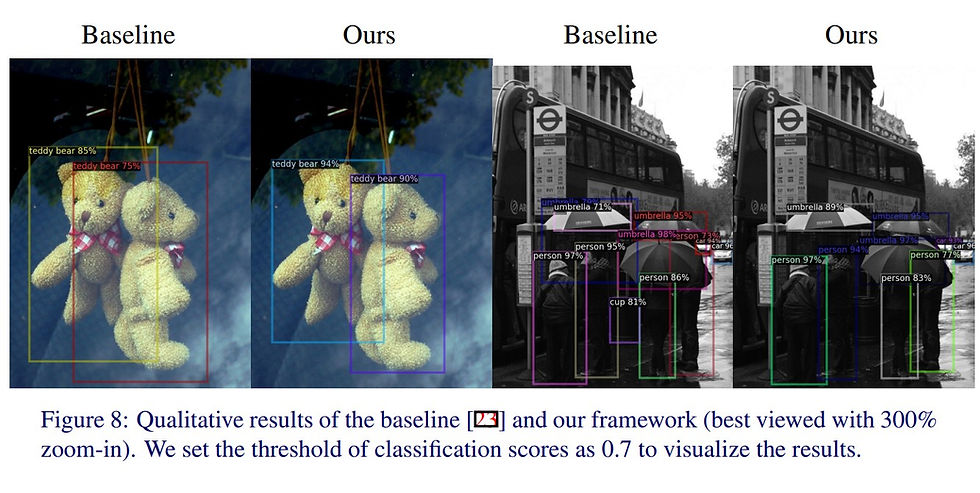
Semi-supervised object detection (SSOD) aims to boost detection performance by leveraging extra unlabeled data.
The teacher-student framework has been shown to be promising for SSOD, in which a teacher network generates pseudo-labels for unlabeled data to assist the training of a student network. Since the pseudo-labels are noisy, filtering the pseudo-labels is crucial
... propose a two-step pseudo-label filtering for the classification and regression heads in a teacher-student framework.
For the classification head, OCL (Object-wise Contrastive Learning) regularizes the object representation learning that utilizes unlabeled data to improve pseudo-label filtering by enhancing the discriminativeness of the classification score.
... For the regression head, ... further propose RUPL (Regression-Uncertainty-guided Pseudo-Labeling) to learn the aleatoric uncertainty of object localization for label filtering.
... demonstrate the superiority of ... proposed method with competitive performance compared to existing methods.
Please like and share this post if you enjoyed it using the buttons at the bottom!
Stay up to date. Subscribe to my posts https://morrislee1234.wixsite.com/website/contact
Web site with my other posts by category https://morrislee1234.wixsite.com/website
Kommentare