Better object detection when train with image-level labels by using synthetic data with D2DF2WOD
- morrislee
- Dec 5, 2022
- 1 min read
Better object detection when train with image-level labels by using synthetic data with D2DF2WOD
D2DF2WOD: Learning Object Proposals for Weakly-Supervised Object Detection via Progressive Domain Adaptation
arXiv paper abstract https://arxiv.org/abs/2212.01376
arXiv PDF paper https://arxiv.org/pdf/2212.01376.pdf
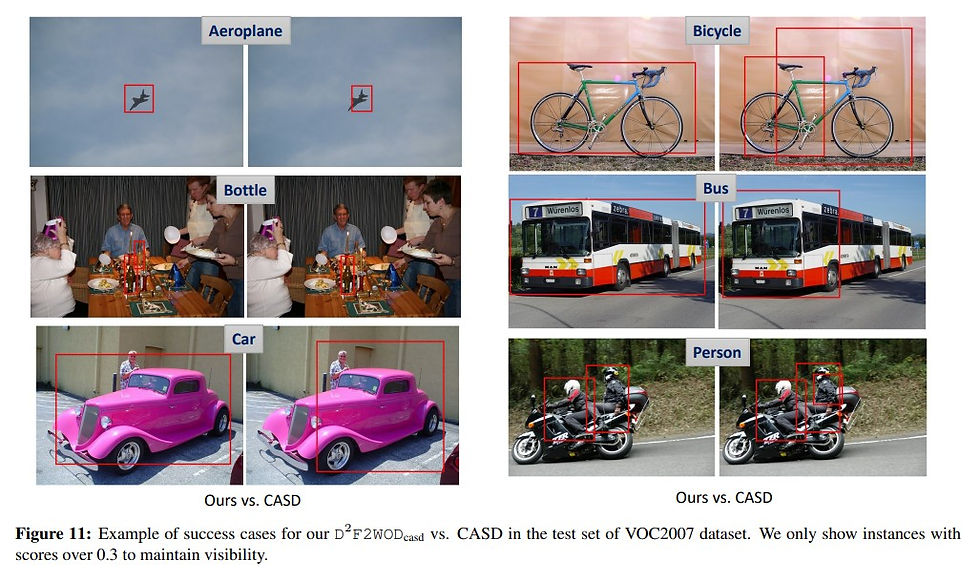
Weakly-supervised object detection (WSOD) models attempt to leverage image-level annotations in lieu of accurate but costly-to-obtain object localization labels.
This oftentimes leads to substandard object detection and localization at inference time.
... propose D2DF2WOD, a Dual-Domain Fully-to-Weakly Supervised Object Detection framework that leverages synthetic data, annotated with precise object localization, to supplement a natural image target domain, where only image-level labels are available.
In its warm-up domain adaptation stage, the model learns a fully-supervised object detector (FSOD) to improve the precision of the object proposals in the target domain, and at the same time learns target-domain-specific and detection-aware proposal features.
In its main WSOD stage, a WSOD model is specifically tuned to the target domain. The feature extractor and the object proposal generator of the WSOD model are built upon the fine-tuned FSOD model.
... method results in consistently improved object detection and localization compared with state-of-the-art methods.
Please like and share this post if you enjoyed it using the buttons at the bottom!
Stay up to date. Subscribe to my posts https://morrislee1234.wixsite.com/website/contact
Web site with my other posts by category https://morrislee1234.wixsite.com/website
Bình luận