Better object detection with semi-supervised training by using offline out-of-domain with Liu
- morrislee
- Aug 30, 2022
- 1 min read
Better object detection with semi-supervised training by using offline out-of-domain with Liu
Open-Set Semi-Supervised Object Detection
arXiv paper abstract https://arxiv.org/abs/2208.13722
arXiv PDF paper https://arxiv.org/pdf/2208.13722.pdf
Project page https://ycliu93.github.io/projects/ossod.html
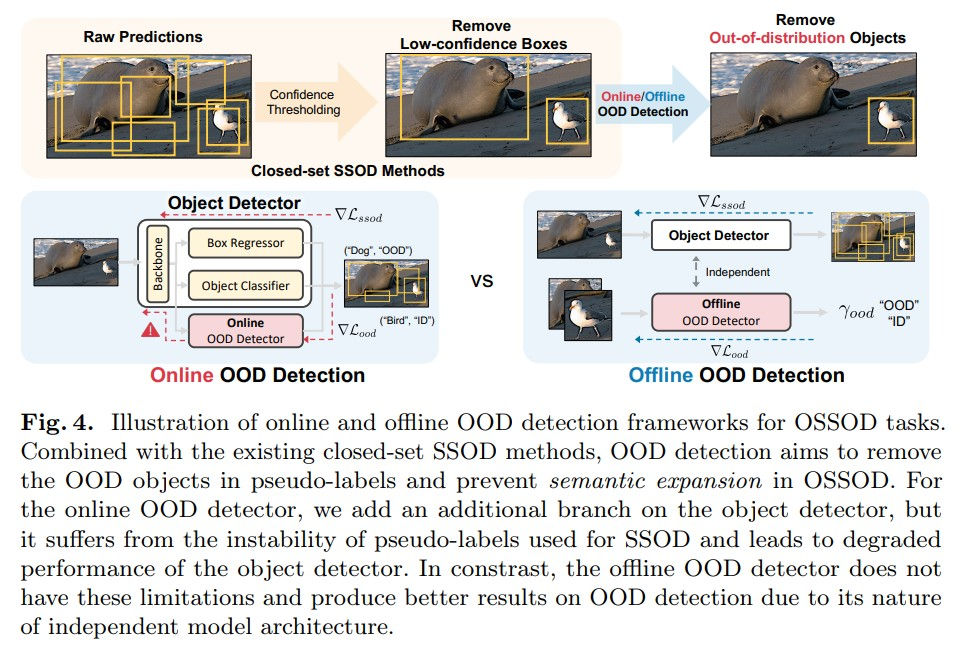
Recent developments for Semi-Supervised Object Detection (SSOD) have shown the promise of leveraging unlabeled data to improve an object detector.
... methods have assumed that the unlabeled data does not contain out-of-distribution (OOD) classes, which is unrealistic with larger-scale unlabeled datasets.
... consider a more practical yet challenging problem, Open-Set Semi-Supervised Object Detection (OSSOD).
... find the existing SSOD method obtains a lower performance gain in open-set conditions, and this is caused by the semantic expansion, where the distracting OOD objects are mispredicted as in-distribution pseudo-labels for the semi-supervised training.
... found that leveraging an offline OOD detector based on a self-supervised vision transformer performs favorably against online OOD detectors due to its robustness to the interference of pseudo-labeling.
... framework effectively addresses the semantic expansion issue and shows consistent improvements on many OSSOD benchmarks, including large-scale COCO-OpenImages ...
Please like and share this post if you enjoyed it using the buttons at the bottom!
Stay up to date. Subscribe to my posts https://morrislee1234.wixsite.com/website/contact
Web site with my other posts by category https://morrislee1234.wixsite.com/website
Comentários