Detect unknown 3D objects in new domains using simulated training data with SemAbs
Detect unknown 3D objects in new domains using simulated training data with SemAbs
Semantic Abstraction: Open-World 3D Scene Understanding from 2D Vision-Language Models
arXiv paper abstract https://arxiv.org/abs/2207.11514
arXiv PDF paper https://arxiv.org/pdf/2207.11514.pdf
Twitter video https://twitter.com/_akhaliq/status/1551738106881212419
Project page https://semantic-abstraction.cs.columbia.edu
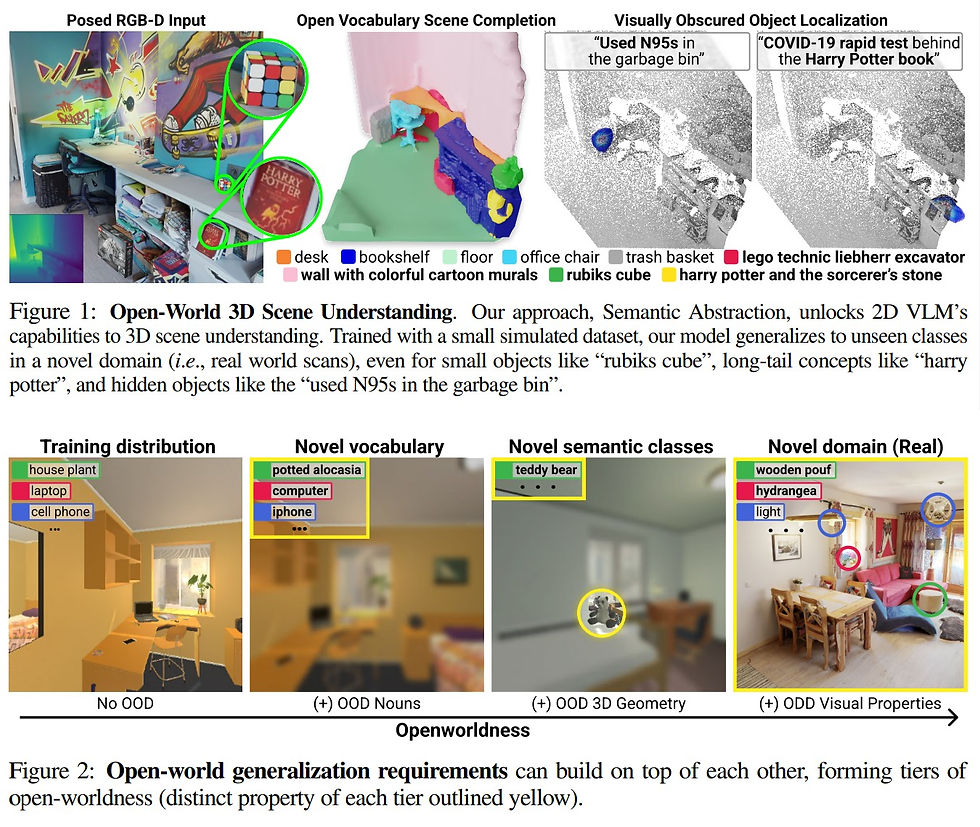
... study open-world 3D scene understanding, a family of tasks that require agents to reason about their 3D environment with an open-set vocabulary and out-of-domain visual inputs - a critical skill for robots to operate in the unstructured 3D world.
... propose Semantic Abstraction (SemAbs), a framework that equips 2D Vision-Language Models (VLMs) with new 3D spatial capabilities, while maintaining their zero-shot robustness.
... achieve this abstraction using relevancy maps extracted from CLIP, and learn 3D spatial and geometric reasoning skills on top of those abstractions in a semantic-agnostic manner.
... demonstrate the usefulness of SemAbs on two open-world 3D scene understanding tasks: 1) completing partially observed objects and 2) localizing hidden objects from language descriptions.
... SemAbs can generalize to novel vocabulary, materials/lighting, classes, and domains (i.e., real-world scans) from training on limited 3D synthetic data ...
Please like and share this post if you enjoyed it using the buttons at the bottom!
Stay up to date. Subscribe to my posts https://morrislee1234.wixsite.com/website/contact
Web site with my other posts by category https://morrislee1234.wixsite.com/website
Comentarios