Find location of image anomalies using neural net trained on simulated anomalies
Updated: Aug 27, 2021
Find location of image anomalies using neural net trained on simulated anomalies
DRÆM -- A discriminatively trained reconstruction embedding for surface anomaly detection
arXiv paper abstract https://arxiv.org/abs/2108.07610v1
arXiv PDF paper https://arxiv.org/pdf/2108.07610v1.pdf
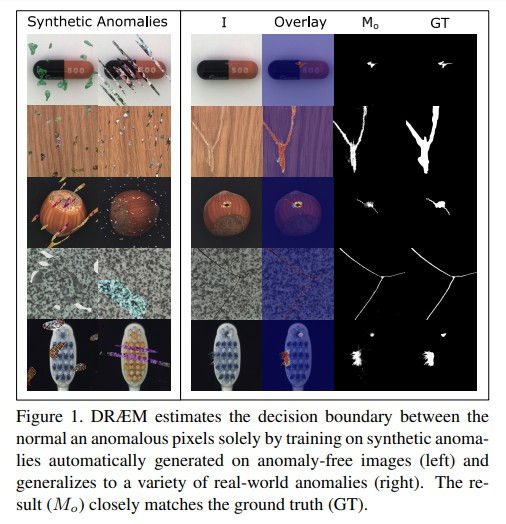
Visual surface anomaly detection aims to detect local image regions that significantly deviate from normal appearance.
Recent surface anomaly detection methods rely on generative models ... trained only on anomaly-free images, and often require hand-crafted post-processing steps to localize the anomalies
... propose ... (DRAEM) ... learns a joint representation of an anomalous image and its anomaly-free reconstruction, while simultaneously learning a decision boundary between normal and anomalous examples.
... enables direct anomaly localization without the need for additional complicated post-processing of the network output and can be trained using simple and general anomaly simulations.
... DRAEM outperforms the current state-of-the-art unsupervised methods by a large margin and even delivers detection performance close to the fully-supervised methods on the widely used DAGM surface-defect detection dataset, while substantially outperforming them in localization accuracy.
Please like and share this post if you enjoyed it using the buttons at the bottom!
Stay up to date. Subscribe to my posts https://morrislee1234.wixsite.com/website/contact
Web site with my other posts by category https://morrislee1234.wixsite.com/website
#ComputerVision #Anomaly #AINewsClips #AI #ML #ArtificialIntelligence #MachineLearning
Comments