Get 3D object shape using neural implicit with self-supervision on surface normals with SN-NIR
- morrislee
- Jun 15, 2024
- 1 min read
Get 3D object shape using neural implicit with self-supervision on surface normals with SN-NIR
Normal-guided Detail-Preserving Neural Implicit Functions for High-Fidelity 3D Surface Reconstruction
arXiv paper abstract https://arxiv.org/abs/2406.04861
arXiv PDF paper https://arxiv.org/pdf/2406.04861
Project page https://sn-nir.github.io
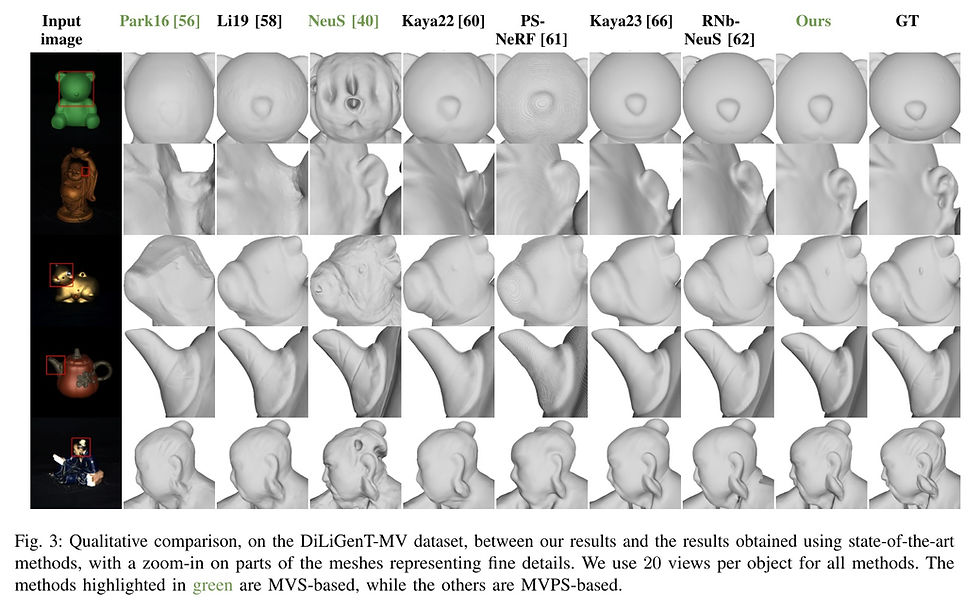
Neural implicit representations ... a powerful paradigm for 3D reconstruction ... fail to capture fine geometric details and thin structures, especially ... where only sparse RGB views
... hypothesize ... methods ... rely on 0-order differential properties, i.e. the 3D surface points and their projections, as supervisory signals. Such properties ... do not capture the local 3D geometry around the points and also ignore the interactions between points.
... training neural representations with first-order differential properties, i.e. surface normals, leads to ... accurate 3D surface reconstruction even ... two RGB (front and back) images
... first compute the approximate surface normals in the image space using the gradient of the depth maps ... using ... monocular depth estimator such as Depth Anything model.
An implicit surface regressor is then trained using a loss function that enforces the first-order differential properties of the regressed surface to match those ... from Depth Anything.
... show that the proposed method achieves an unprecedented level of reconstruction accuracy even when using as few as two RGB views ...
Please like and share this post if you enjoyed it using the buttons at the bottom!
Stay up to date. Subscribe to my posts https://morrislee1234.wixsite.com/website/contact
Web site with my other posts by category https://morrislee1234.wixsite.com/website
Comentarios