Get absolute depth of scene from monocular image by diffusion, field-of-view, augmentation with DMD
- morrislee
- Dec 21, 2023
- 1 min read
Get absolute depth of scene from monocular image by diffusion, field-of-view, augmentation with DMD
Zero-Shot Metric Depth with a Field-of-View Conditioned Diffusion Model
arXiv paper abstract https://arxiv.org/abs/2312.13252
arXiv PDF paper https://arxiv.org/pdf/2312.13252.pdf
Project page https://diffusion-vision.github.io/dmd
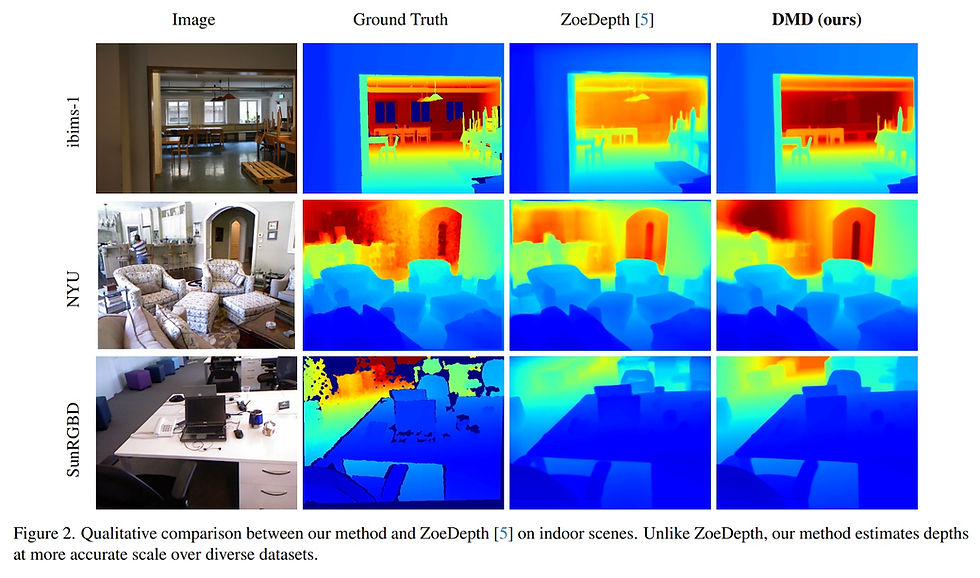
While methods for monocular depth estimation have made significant strides on standard benchmarks, zero-shot metric depth estimation remains unsolved.
Challenges include the joint modeling of indoor and outdoor scenes, which often exhibit significantly different distributions of RGB and depth, and the depth-scale ambiguity due to unknown camera intrinsics.
Recent work has proposed specialized multi-head architectures for jointly modeling indoor and outdoor scenes.
... advocate a generic, task-agnostic diffusion model, with several advancements such as log-scale depth parameterization to enable joint modeling of indoor and outdoor scenes, conditioning on the field-of-view (FOV) to handle scale ambiguity and synthetically augmenting FOV during training to generalize beyond the limited camera intrinsics in training datasets.
... by employing a more diverse training mixture ... and an efficient diffusion parameterization, ... method, DMD (Diffusion for Metric Depth) achieves ... reduction in relative error ... on ... indoor and ... outdoor ... over the current SOTA ...
Please like and share this post if you enjoyed it using the buttons at the bottom!
Stay up to date. Subscribe to my posts https://morrislee1234.wixsite.com/website/contact
Web site with my other posts by category https://morrislee1234.wixsite.com/website
Comments