Improve 3D object detection by using 3D equivariant neural network with DEVIANT
Improve 3D object detection by using 3D equivariant neural network with DEVIANT
DEVIANT: Depth EquiVarIAnt NeTwork for Monocular 3D Object Detection
arXiv paper abstract https://arxiv.org/abs/2207.10758
arXiv PDF paper https://arxiv.org/pdf/2207.10758.pdf
Twitter video https://twitter.com/_akhaliq/status/1551428840324354048
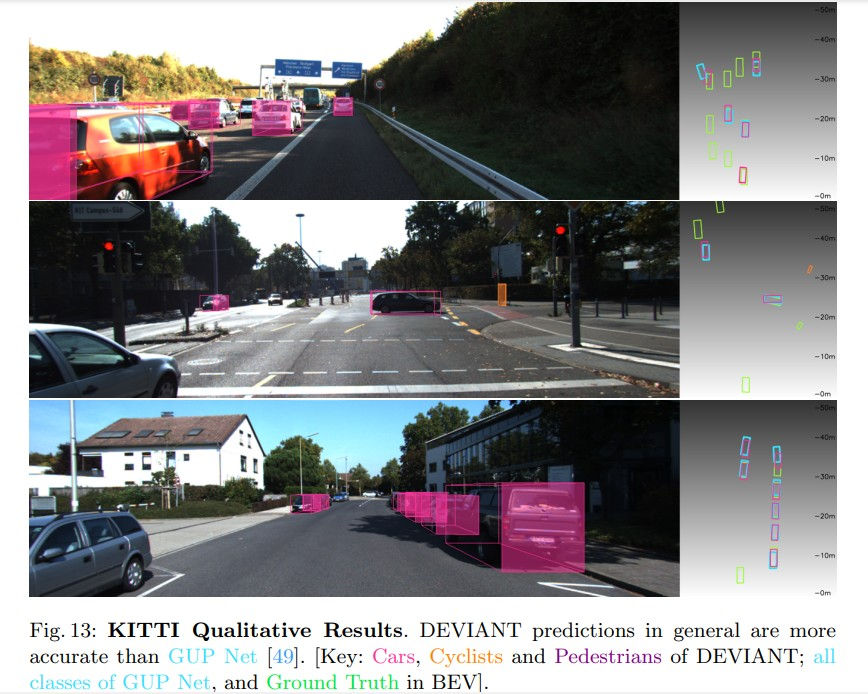
Modern neural networks use building blocks such as convolutions that are equivariant to arbitrary 2D translations.
However, these vanilla blocks are not equivariant to arbitrary 3D translations in the projective manifold.
... paper takes the first step towards convolutions equivariant to arbitrary 3D translations in the projective manifold.
... proposes Depth EquiVarIAnt NeTwork (DEVIANT) built with existing scale equivariant steerable blocks.
... DEVIANT is equivariant to the depth translations in the projective manifold whereas vanilla networks are not.
... achieves state-of-the-art monocular 3D detection results on KITTI and Waymo datasets in the image-only category and performs competitively to methods using extra information ...
Please like and share this post if you enjoyed it using the buttons at the bottom!
Stay up to date. Subscribe to my posts https://morrislee1234.wixsite.com/website/contact
Web site with my other posts by category https://morrislee1234.wixsite.com/website
コメント