Improve depth resolution at any scaling factor using geometric information with GeoDSR
Improve depth resolution at any scaling factor using geometric information with GeoDSR
Learning Continuous Depth Representation via Geometric Spatial Aggregator
arXiv paper abstract https://arxiv.org/abs/2212.03499
arXiv PDF paper https://arxiv.org/pdf/2212.03499.pdf
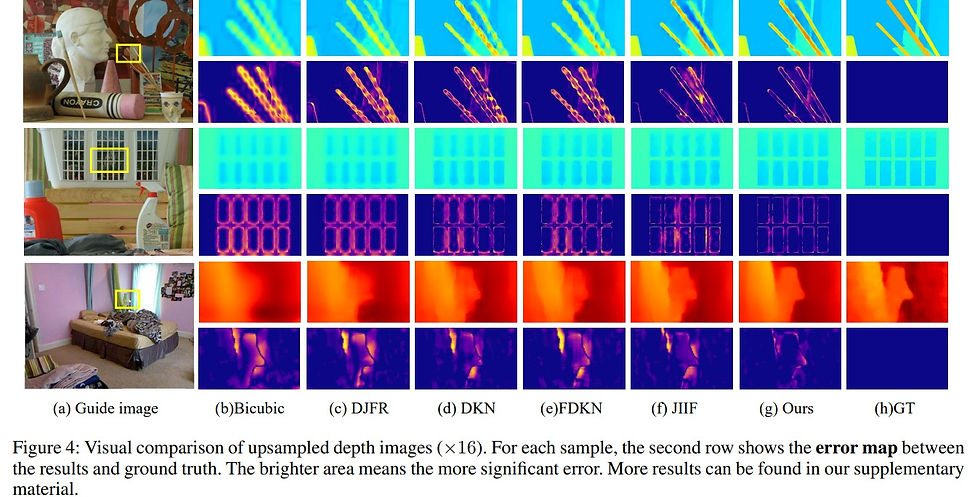
Depth map super-resolution (DSR) has been a fundamental task for 3D computer vision.
While arbitrary scale DSR is a more realistic setting in this scenario, previous approaches predominantly suffer from the issue of inefficient real-numbered scale upsampling.
... propose a novel continuous depth representation for DSR.
... proposed Geometric Spatial Aggregator (GSA), which exploits a distance field modulated by arbitrarily upsampled target gridding, through which the geometric information is explicitly introduced into feature aggregation and target generation.
... present a transformer-style backbone named GeoDSR, which possesses a principled way to construct the functional mapping between local coordinates and the high-resolution output results, empowering ... model with the advantage of arbitrary shape transformation ready to help diverse zooming demand.
... framework achieves significant restoration gain in arbitrary scale depth map super-resolution compared with the prior art ...
Please like and share this post if you enjoyed it using the buttons at the bottom!
Stay up to date. Subscribe to my posts https://morrislee1234.wixsite.com/website/contact
Web site with my other posts by category https://morrislee1234.wixsite.com/website
LinkedIn https://www.linkedin.com/in/morris-lee-47877b7b
#ComputerVision #3D #AINewsClips #AI #ML #ArtificialIntelligence #MachineLearning
Kommentare