Improve image segmentation by identifying and relabeling out-of-candidate pixels with OCR
Improve image segmentation by identifying and relabeling out-of-candidate pixels with OCR
Out-of-Candidate Rectification for Weakly Supervised Semantic Segmentation
arXiv paper abstract https://arxiv.org/abs/2211.12268
arXiv PDF paper https://arxiv.org/pdf/2211.12268.pdf
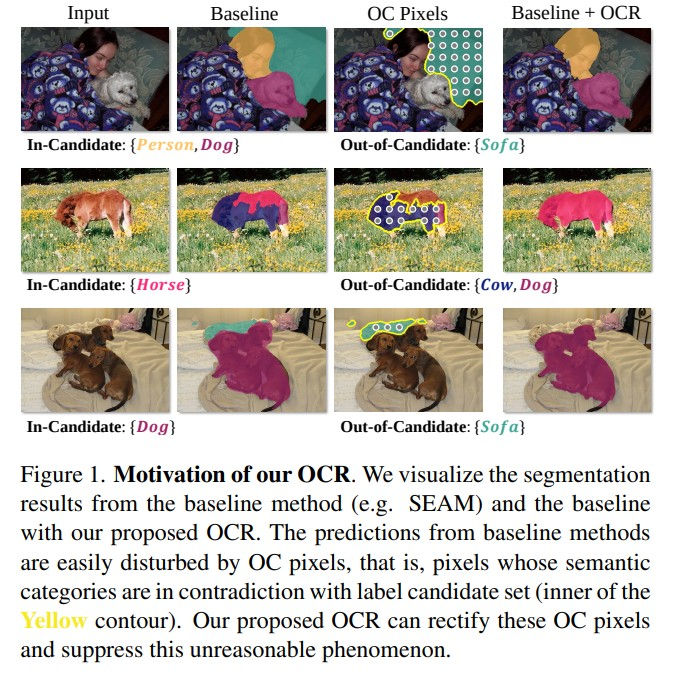
Weakly supervised semantic segmentation is typically inspired by class activation maps, which serve as pseudo masks with class-discriminative regions highlighted.
... existing methods still commonly suffer from the unsolicited Out-of-Candidate (OC) error predictions that not belongs to the label candidates, which could be avoidable since the contradiction with image-level class tags is easy to be detected.
... develop a group ranking-based Out-of-Candidate Rectification (OCR) mechanism in a plug-and-play fashion.
Firstly, ... adaptively split the semantic categories into In-Candidate (IC) and OC groups for each OC pixel according to their prior annotation correlation and posterior prediction correlation.
Then, ... derive a differentiable rectification loss to force OC pixels to shift to the IC group.
... OCR ... can achieve remarkable performance gains on both Pascal VOC ... and MS COCO ... datasets with negligible extra training overhead ...
Please like and share this post if you enjoyed it using the buttons at the bottom!
Stay up to date. Subscribe to my posts https://morrislee1234.wixsite.com/website/contact
Web site with my other posts by category https://morrislee1234.wixsite.com/website
Comentários