Improve object detection under difficult conditions by learning many image pre-processors with GDIP
- morrislee
- Oct 5, 2022
- 1 min read
Improve object detection under difficult conditions by learning many image pre-processors with GDIP
GDIP: Gated Differentiable Image Processing for Object-Detection in Adverse Conditions
arXiv paper abstract https://arxiv.org/abs/2209.14922v1
arXiv PDF paper https://arxiv.org/pdf/2209.14922v1.pdf
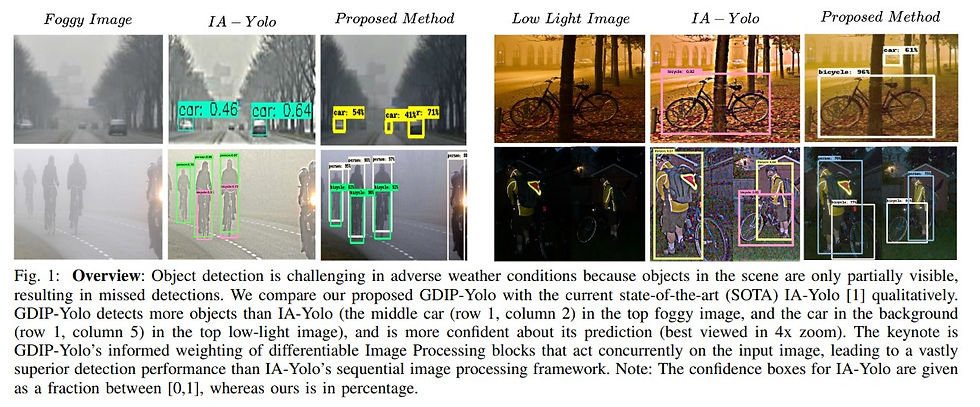
Detecting objects under adverse weather and lighting conditions is crucial for the safe and continuous operation of an autonomous vehicle, and remains an unsolved problem.
... present a Gated Differentiable Image Processing (GDIP) block, a domain-agnostic network architecture, which can be plugged into existing object detection networks (e.g., Yolo) and trained end-to-end with adverse condition images such as those captured under fog and low lighting.
... proposed GDIP block learns to enhance images directly through the downstream object detection loss.
... achieved by learning parameters of multiple image pre-processing (IP) techniques that operate concurrently, with their outputs combined using weights learned through a novel gating mechanism.
... trading off accuracy for speed ... propose a variant of GDIP that can be used as a regularizer for training Yolo, which eliminates the need for GDIP-based image enhancement during inference, resulting in higher throughput and plausible real-world deployment.
... demonstrate significant improvement in detection performance over several state-of-the-art methods ...
Please like and share this post if you enjoyed it using the buttons at the bottom!
Stay up to date. Subscribe to my posts https://morrislee1234.wixsite.com/website/contact
Web site with my other posts by category https://morrislee1234.wixsite.com/website
Comments