Improve scene segmentation with smaller models by distilling knowledge with SKR+PEA
- morrislee
- Mar 2, 2022
- 1 min read
Improve scene segmentation with smaller models by distilling knowledge with SKR+PEA
Transformer-based Knowledge Distillation for Efficient Semantic Segmentation of Road-driving Scenes
arXiv paper abstract https://arxiv.org/abs/2202.13393v1
arXiv PDF paper https://arxiv.org/pdf/2202.13393v1.pdf
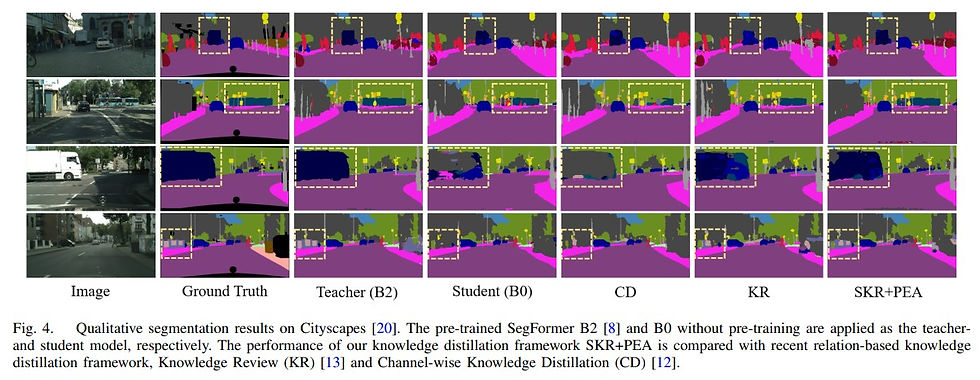
For scene understanding in robotics and automated driving, there is ... interest in solving semantic segmentation tasks with transformer-based methods.
However, effective transformers are always too cumbersome and computationally expensive to solve semantic segmentation in real time
... Knowledge Distillation (KD) speeds up inference and maintains accuracy while transferring knowledge from a pre-trained cumbersome teacher model to a compact student model.
... present a novel KD framework ... training compact transformers by transferring the knowledge from feature maps and patch embeddings of large transformers.
... two modules ... proposed: (1) ... an efficient relation-based KD framework ... (SKR); (2) ... (PEA) ... performs the dimensional transformation of patch embeddings. The combined KD framework is called SKR+PEA.
... approach outperforms recent state-of-the-art KD frameworks and rivals the time-consuming pre-training method. ...
Please like and share this post if you enjoyed it using the buttons at the bottom!
Stay up to date. Subscribe to my posts https://morrislee1234.wixsite.com/website/contact
Web site with my other posts by category https://morrislee1234.wixsite.com/website
Comments