Learn new objects without forgetting old ones with transformers by using reliability with CL-DETR
Learn new objects without forgetting old ones with transformers by using reliability with CL-DETR
Continual Detection Transformer for Incremental Object Detection
arXiv paper abstract https://arxiv.org/abs/2304.03110
arXiv PDF paper https://arxiv.org/pdf/2304.03110.pdf
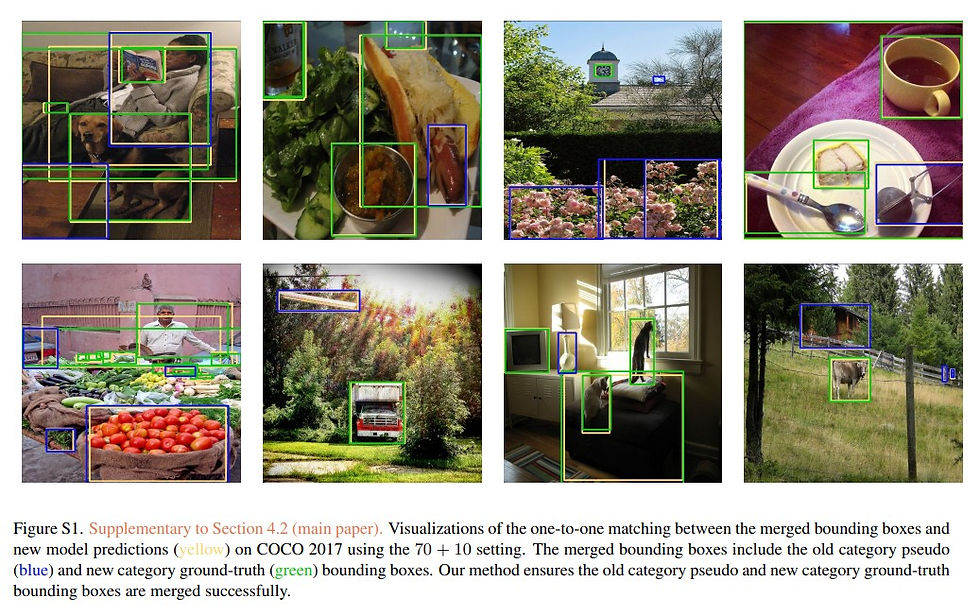
Incremental object detection (IOD) aims to train an object detector in phases, each with annotations for new object categories.
... incremental ... IOD is subject to catastrophic forgetting, which is often addressed by techniques such as knowledge distillation (KD) and exemplar replay (ER). However, KD and ER do not work well if applied directly to ... transformer-based object detectors ...
... solve these issues by proposing a ContinuaL DEtection TRansformer (CL-DETR), a new method for transformer-based IOD which enables effective usage of KD and ER in this context.
... introduce a Detector Knowledge Distillation (DKD) loss, focusing on the most informative and reliable predictions from old versions of the model, ignoring redundant background predictions, and ensuring compatibility with the available ground-truth labels.
... improve ER by proposing a calibration strategy to preserve the label distribution of the training set, therefore better matching training and testing statistics.
... demonstrate that CL-DETR achieves state-of-the-art results in the IOD setting.
Please like and share this post if you enjoyed it using the buttons at the bottom!
Stay up to date. Subscribe to my posts https://morrislee1234.wixsite.com/website/contact
Web site with my other posts by category https://morrislee1234.wixsite.com/website
Comments