Monocular 3D depth for edge device with self-supervised learn by CNN and transformers with Lite-Mono
Monocular 3D depth for edge device with self-supervised learn by CNN and transformers with Lite-Mono
Lite-Mono: A Lightweight CNN and Transformer Architecture for Self-Supervised Monocular Depth Estimation
arXiv paper abstract https://arxiv.org/abs/2211.13202
arXiv PDF paper https://arxiv.org/pdf/2211.13202.pdf
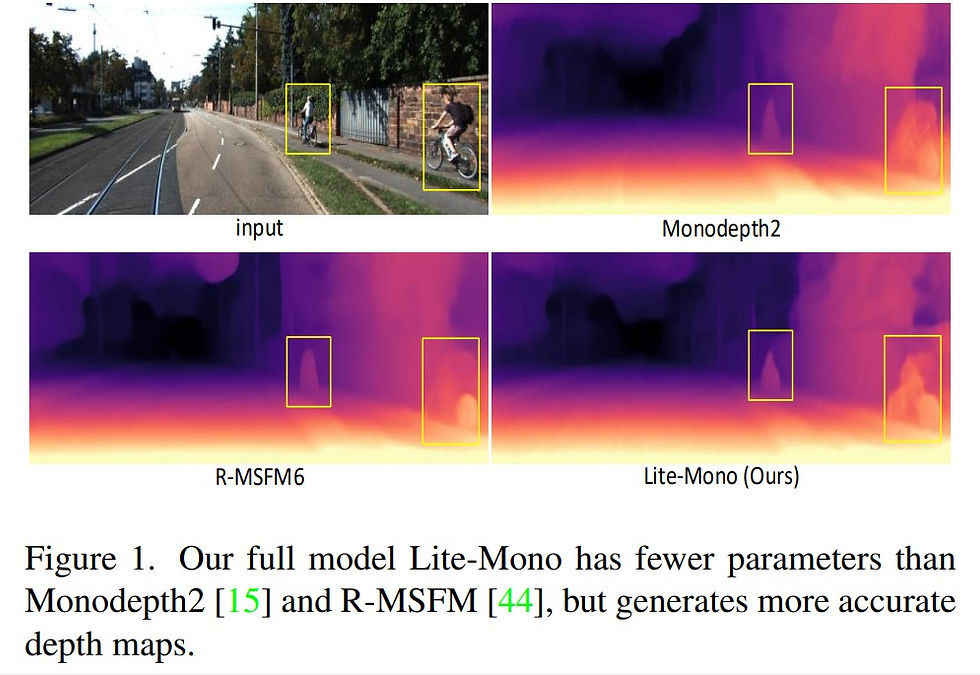
Self-supervised monocular depth estimation that does not require ground-truth for training has attracted attention in recent years.
It is of high interest to design lightweight but effective models, so that they can be deployed on edge devices.
... In this paper ... achieve comparable results with a lightweight architecture.
Specifically, ... investigate the efficient combination of CNNs and Transformers, and design a hybrid architecture Lite-Mono.
... The former is used to extract rich multi-scale local features, and the latter takes advantage of the self-attention mechanism to encode long-range global information into the features.
... demonstrate that ... full model outperforms Monodepth2 by a large margin in accuracy, with about 80% fewer trainable parameters.
Please like and share this post if you enjoyed it using the buttons at the bottom!
Stay up to date. Subscribe to my posts https://morrislee1234.wixsite.com/website/contact
Web site with my other posts by category https://morrislee1234.wixsite.com/website
تعليقات