NVIDIA, Stanford, Microsoft Efficient Trillion-Parameter Model Training on GPU Clusters
NVIDIA, Stanford, Microsoft Efficient Trillion-Parameter Model Training on GPU Clusters
NVIDIA, Stanford & Microsoft Propose Efficient Trillion-Parameter Language Model Training on GPU Clusters
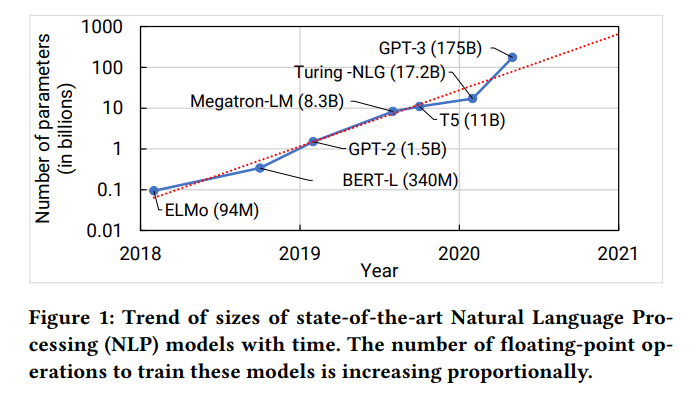
Efficient Large-Scale Language Model Training on GPU Clusters
arXiv paper abstract https://arxiv.org/abs/2104.04473?context=cs.CL
arXiv PDF paper https://arxiv.org/pdf/2104.04473.pdf
... In this work, we show how to compose different types of parallelism methods (tensor, pipeline, and data paralleism) to scale to thousands of GPUs, achieving a two-order-of-magnitude increase in the sizes of models we can efficiently train compared to existing systems. ... The composition of these techniques allows us to perform training iterations on a model with 1 trillion parameters at 502 petaFLOP/s on 3072 GPUs with achieved per-GPU throughput of 52% of peak; previous efforts to train similar-sized models achieve much lower throughput (36% of theoretical peak).
Please like and share this post if you enjoyed it using the buttons at the bottom!
Stay up to date. Subscribe to my posts https://morrislee1234.wixsite.com/website/contact
Web site with my other posts by category https://morrislee1234.wixsite.com/website
Comments