Predict future depth and motion using only self-supervised raw images as input
Predict future depth and motion using only self-supervised raw images as input
Forecasting of depth and ego-motion with transformers and self-supervision
arXiv paper abstract https://arxiv.org/abs/2206.07435v1
arXiv PDF paper https://arxiv.org/pdf/2206.07435v1.pdf
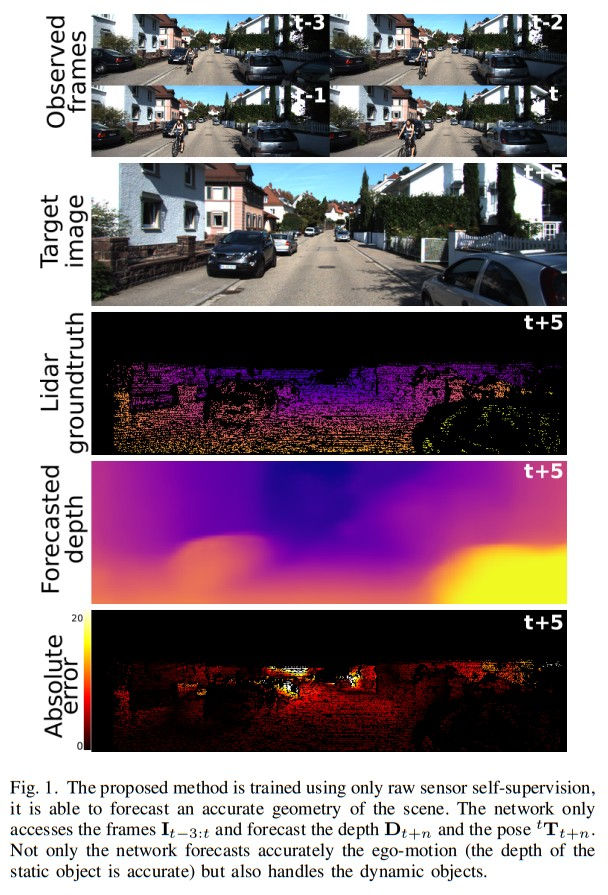
... paper addresses the problem of end-to-end self-supervised forecasting of depth and ego motion.
Given a sequence of raw images, the aim is to forecast both the geometry and ego-motion using a self supervised photometric loss.
The architecture is designed using both convolution and transformer modules.
... leverages the benefits of both modules: Inductive bias of CNN, and the multi-head attention of transformers, thus enabling a rich spatio-temporal representation that enables accurate depth forecasting.
... paper forecasts depth and ego motion using only self-supervised raw images as input.
... performs significantly well on the KITTI dataset benchmark with several performance criteria being even comparable to prior non-forecasting self-supervised monocular depth inference methods
Please like and share this post if you enjoyed it using the buttons at the bottom!
Stay up to date. Subscribe to my posts https://morrislee1234.wixsite.com/website/contact
Web site with my other posts by category https://morrislee1234.wixsite.com/website
Comments