Put partial 3D point clouds into standard orientation with self-supervised ConDor
- morrislee
- Jan 25, 2022
- 1 min read
Updated: Apr 25, 2022
Put partial 3D point clouds into standard orientation with self-supervised ConDor
ConDor: Self-Supervised Canonicalization of 3D Pose for Partial Shapes
arXiv paper abstract https://arxiv.org/abs/2201.07788
arXiv PDF paper https://arxiv.org/pdf/2201.07788.pdf
Project page https://ivl.cs.brown.edu/ConDor
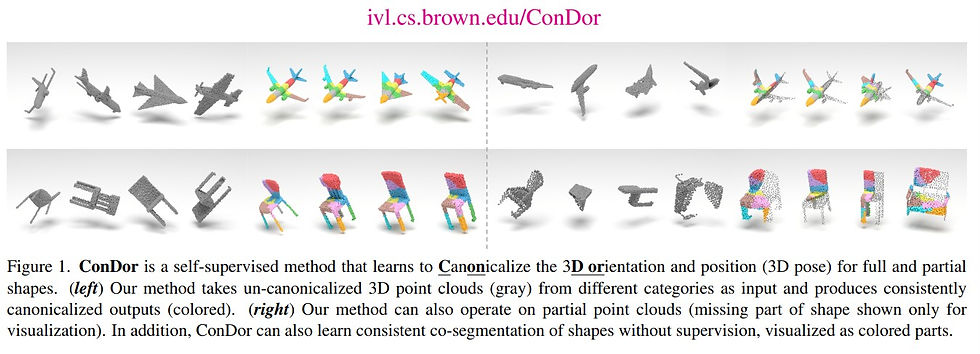
Progress in 3D object understanding has relied on manually canonicalized shape datasets that contain instances with consistent position and orientation (3D pose).
... ConDor is a self-supervised method that learns to Canonicalize the 3D orientation and position for full and partial 3D point clouds.
... build on top of Tensor Field Networks (TFNs) ... method takes an unseen full or partial 3D point cloud at an arbitrary pose and outputs an equivariant canonical pose.
... network uses self-supervision losses to learn the canonical pose from an un-canonicalized collection of full and partial 3D point clouds.
ConDor can also learn to consistently co-segment object parts without any supervision.
... approach outperforms existing methods while enabling new applications such as operation on depth images and annotation transfer.
Please like and share this post if you enjoyed it using the buttons at the bottom!
Stay up to date. Subscribe to my posts https://morrislee1234.wixsite.com/website/contact
Web site with my other posts by category https://morrislee1234.wixsite.com/website
#ComputerVision #3D #AINewsClips #AI #ML #ArtificialIntelligence #MachineLearning
Komentar