Segment and complete 3D scene with unsupervised learning by fusing cross-domain features with S4R
- morrislee
- Feb 8, 2023
- 1 min read
Segment and complete 3D scene with unsupervised learning by fusing cross-domain features with S4R
S4R: Self-Supervised Semantic Scene Reconstruction from RGB-D Scans
arXiv paper abstract https://arxiv.org/abs/2302.03640
arXiv PDF paper https://arxiv.org/pdf/2302.03640.pdf
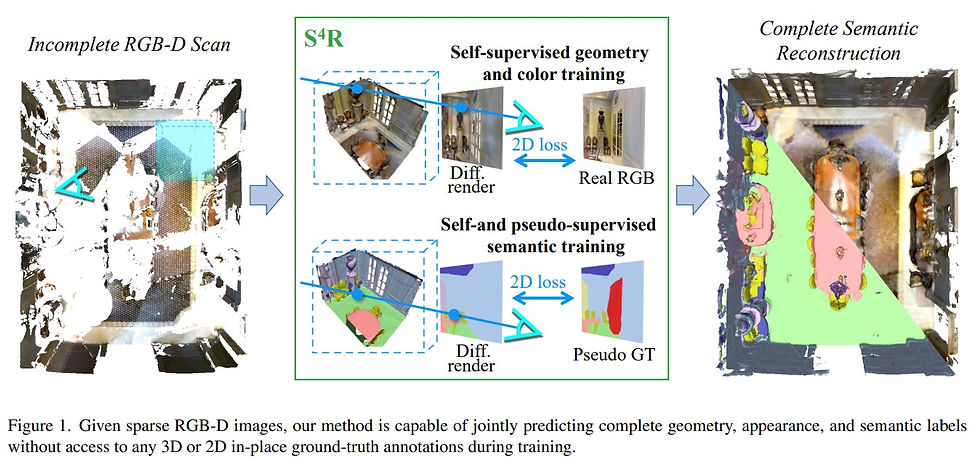
Most deep learning approaches to comprehensive semantic modeling of 3D indoor spaces require costly dense annotations in the 3D domain.
... explore a central 3D scene modeling task, namely, semantic scene reconstruction, using a fully self-supervised approach.
... design a trainable model that employs both incomplete 3D reconstructions and their corresponding source RGB-D images, fusing cross-domain features into volumetric embeddings to predict complete 3D geometry, color, and semantics.
... propose an end-to-end trainable solution jointly addressing geometry completion, colorization, and semantic mapping from a few RGB-D images, without 3D or 2D ground-truth.
... method is the first ... fully self-supervised method addressing completion and semantic segmentation of real-world 3D scans.
... performs comparably well with the 3D supervised baselines, surpasses baselines with 2D supervision on real datasets, and generalizes well to unseen scenes.
Please like and share this post if you enjoyed it using the buttons at the bottom!
Stay up to date. Subscribe to my posts https://morrislee1234.wixsite.com/website/contact
Web site with my other posts by category https://morrislee1234.wixsite.com/website
Comments