Segment scene using vision-language models for diverse semantic knowledge with SemiVL
Segment scene using vision-language models for diverse semantic knowledge with SemiVL
SemiVL: Semi-Supervised Semantic Segmentation with Vision-Language Guidance
arXiv paper abstract https://arxiv.org/abs/2311.16241
arXiv PDF paper https://arxiv.org/pdf/2311.16241.pdf
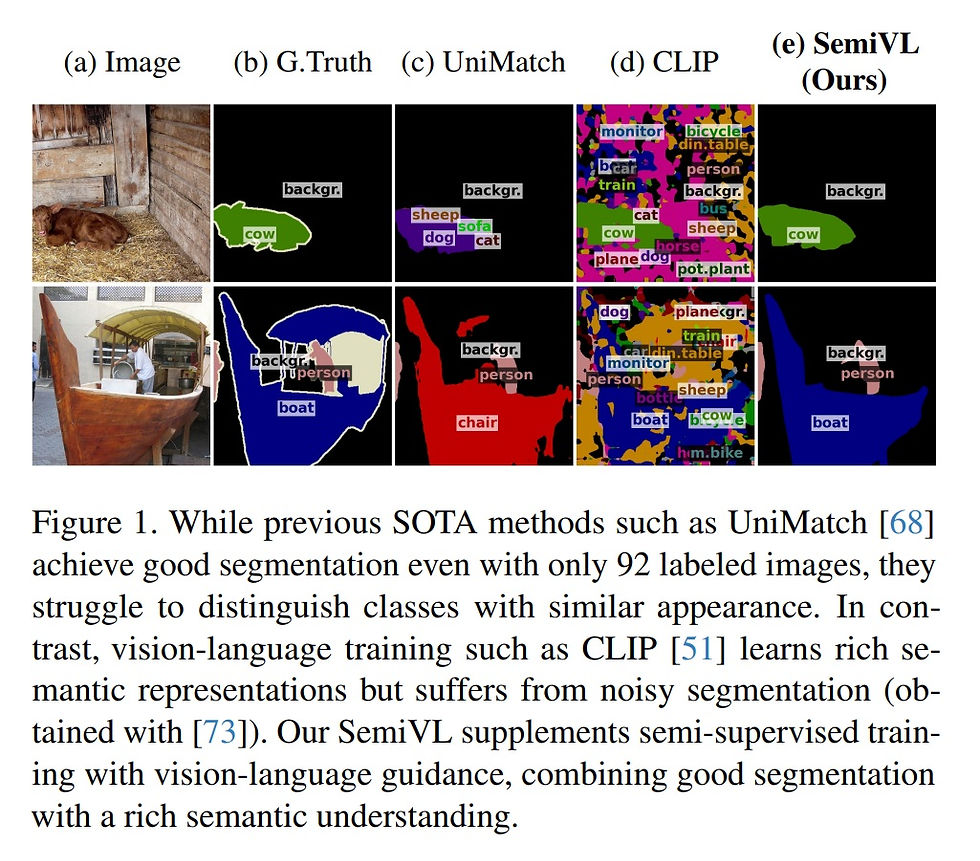
In semi-supervised semantic segmentation, a model is trained with a limited number of labeled images along with a large corpus of unlabeled images to reduce the high annotation effort.
While previous methods are able to learn good segmentation boundaries, they are prone to confuse classes with similar visual appearance due to the limited supervision.
On the other hand, vision-language models (VLMs) are able to learn diverse semantic knowledge from image-caption datasets but produce noisy segmentation due to the image-level training.
In SemiVL, ... propose to integrate rich priors from VLM pre-training into semi-supervised semantic segmentation to learn better semantic decision boundaries.
To adapt the VLM from global to local reasoning, ... introduce a spatial fine-tuning strategy for label-efficient learning ... design a language-guided decoder to jointly reason over vision and language.
... SemiVL ... significantly outperforms previous semi-supervised methods ...
Please like and share this post if you enjoyed it using the buttons at the bottom!
Stay up to date. Subscribe to my posts https://morrislee1234.wixsite.com/website/contact
Web site with my other posts by category https://morrislee1234.wixsite.com/website
Commentaires