Segment scene with SAM efficiently by change bimodal distribution to quantized normal with PTQ4SAM
Segment scene with SAM efficiently by change bimodal distribution to quantized normal with PTQ4SAM
PTQ4SAM: Post-Training Quantization for Segment Anything
arXiv paper abstract https://arxiv.org/abs/2405.03144
arXiv PDF paper https://arxiv.org/pdf/2405.03144
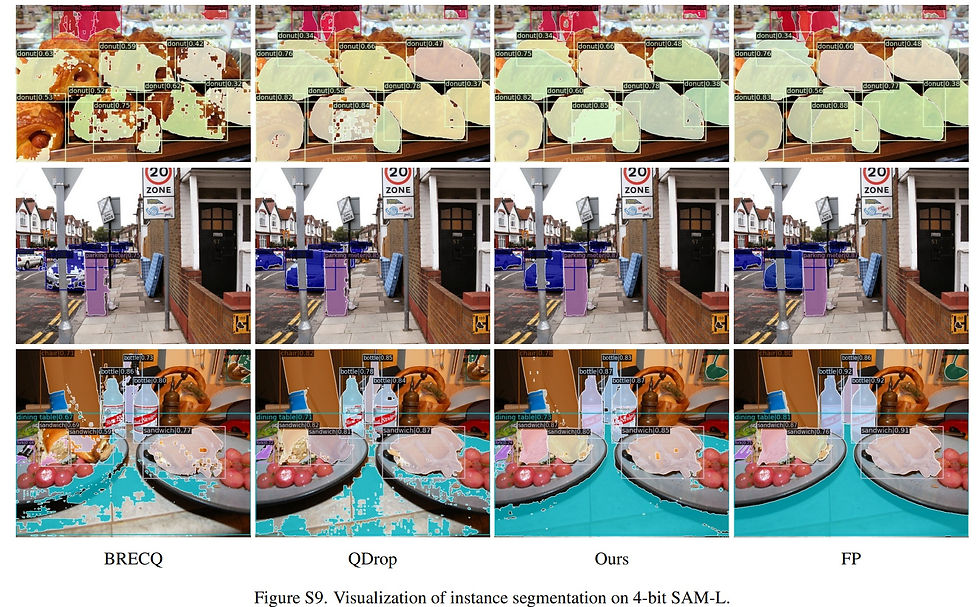
Segment Anything Model (SAM) has ... impressive performance ... However ... immense memory and computation costs hinder its practical deployment.
... propose a post-training quantization (PTQ) framework for Segment Anything Model, namely PTQ4SAM ... investigate ... bottleneck of SAM quantization attributed to the bimodal distribution in post-Key-Linear activations.
... analyze its characteristics from ... per-tensor and per-channel perspectives, and propose a Bimodal Integration strategy, which utilizes a mathematically equivalent sign operation to transform the bimodal distribution into ... easy-quantized normal distribution offline.
Second, SAM encompasses diverse attention mechanisms (i.e., self-attention and two-way cross-attention), resulting in substantial variations in the post-Softmax distributions.
Therefore, ... introduce an Adaptive Granularity Quantization for Softmax through searching the optimal power-of-two base, which is hardware-friendly.
... results across ... instance segmentation, semantic segmentation and object detection .. datasets and model variants show the superiority of PTQ4SAM ...
Please like and share this post if you enjoyed it using the buttons at the bottom!
Stay up to date. Subscribe to my posts https://morrislee1234.wixsite.com/website/contact
Web site with my other posts by category https://morrislee1234.wixsite.com/website
Comments