Segment scene with unknown objects without training by diffusion for reference embedding with FreeDA
Segment scene with unknown objects without training by diffusion for reference embedding with FreeDA
Training-Free Open-Vocabulary Segmentation with Offline Diffusion-Augmented Prototype Generation
arXiv paper abstract https://arxiv.org/abs/2404.06542
arXiv PDF paper https://arxiv.org/pdf/2404.06542.pdf
Project page https://aimagelab.github.io/freeda
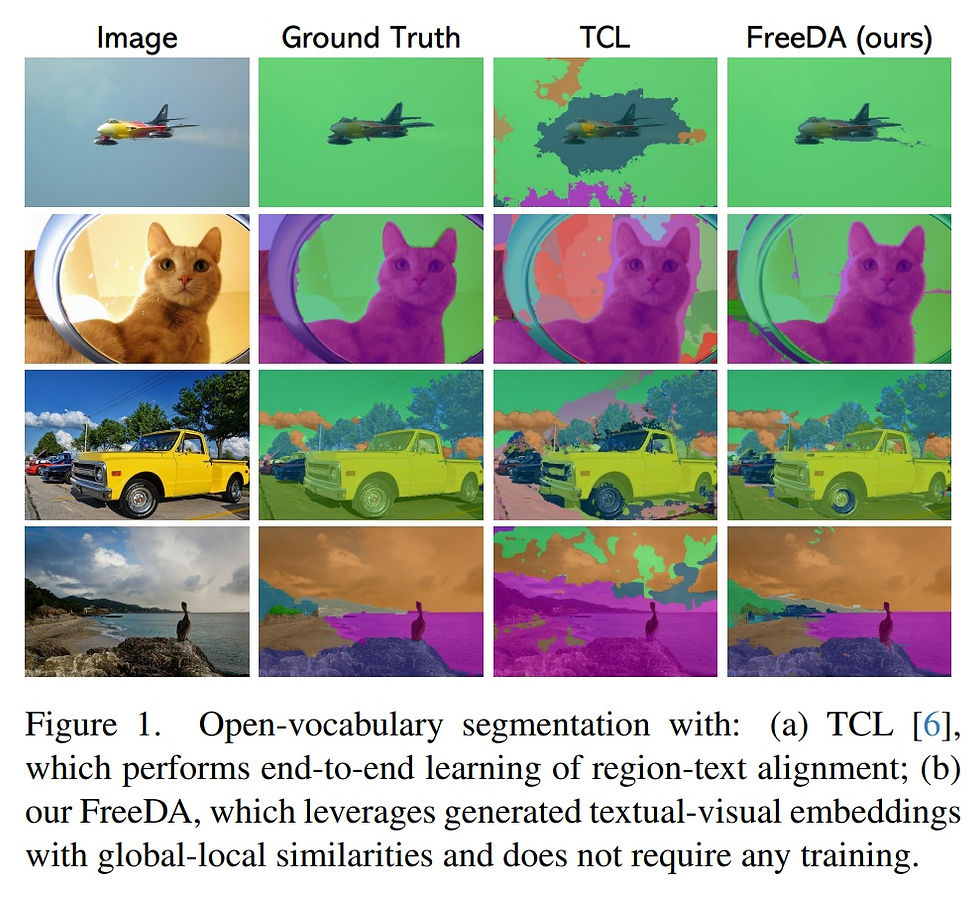
Open-vocabulary semantic segmentation aims at segmenting arbitrary categories expressed in textual form.
Previous ... trained over large amounts of image-caption pairs ... However, captions ... lack localization of ... concepts ... training on large-scale datasets ... brings ... costs.
... propose FreeDA, a training-free diffusion-augmented method for open-vocabulary semantic segmentation, which leverages the ability of diffusion models to visually localize generated concepts and local-global similarities to match class-agnostic regions with semantic classes.
... approach involves an offline stage in which textual-visual reference embeddings are collected, starting from a large set of captions and leveraging visual and semantic contexts.
At test time, these are queried to support the visual matching process, which is carried out by jointly considering class-agnostic regions and global semantic similarities.
... demonstrate that FreeDA achieves state-of-the-art performance on five datasets, surpassing previous methods ... without requiring any training.
Please like and share this post if you enjoyed it using the buttons at the bottom!
Stay up to date. Subscribe to my posts https://morrislee1234.wixsite.com/website/contact
Web site with my other posts by category https://morrislee1234.wixsite.com/website
Comments