Segmentation unknown object using unsupervised learning and stable diffusion with DiffSeg
Segmentation unknown object using unsupervised learning and stable diffusion with DiffSeg
Diffuse, Attend, and Segment: Unsupervised Zero-Shot Segmentation using Stable Diffusion
arXiv paper abstract https://arxiv.org/abs/2308.12469
arXiv PDF paper https://arxiv.org/pdf/2308.12469.pdf
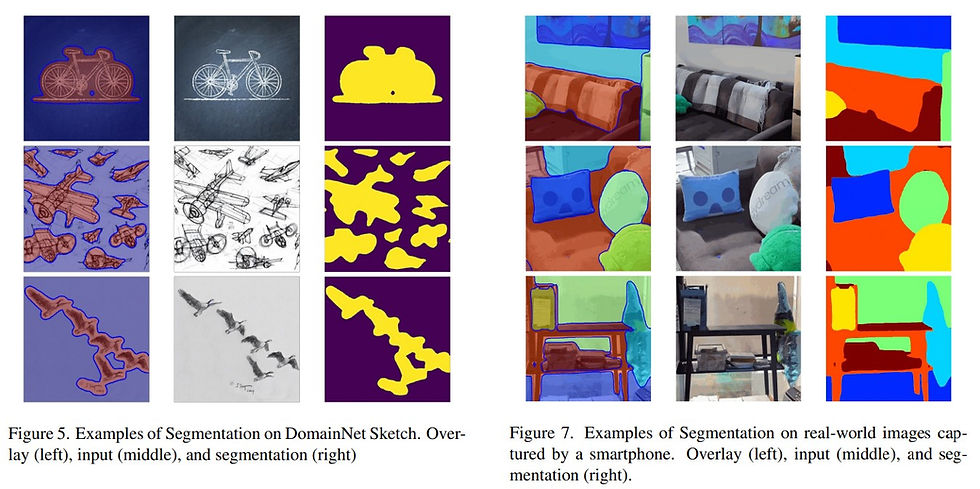
Producing quality segmentation masks for images is a fundamental problem in computer vision.
... However, constructing a model capable of segmenting anything in a zero-shot manner without any annotations is still challenging.
... propose to utilize the self-attention layers in stable diffusion models to achieve this goal because the pre-trained stable diffusion model has learned inherent concepts of objects within its attention layers.
... introduce a simple yet effective iterative merging process based on measuring KL divergence among attention maps to merge them into valid segmentation masks.
The proposed method does not require any training or language dependency to extract quality segmentation for any images.
... method surpasses the prior unsupervised zero-shot SOTA method by an absolute 26% in pixel accuracy and 17% in mean IoU.
Please like and share this post if you enjoyed it using the buttons at the bottom!
Stay up to date. Subscribe to my posts https://morrislee1234.wixsite.com/website/contact
Web site with my other posts by category https://morrislee1234.wixsite.com/website
LinkedIn https://www.linkedin.com/in/morris-lee-47877b7b
#ComputerVision #Segmentation #AINewsClips #AI #ML #ArtificialIntelligence #MachineLearning
Comments