Semi-supervised segmentation using learning with augmentation in image and feature space with DSSN
Semi-supervised segmentation using learning with augmentation in image and feature space with DSSN
Improving Semi-Supervised Semantic Segmentation with Dual-Level Siamese Structure Network
arXiv paper abstract https://arxiv.org/abs/2307.13938
arXiv PDF paper https://arxiv.org/pdf/2307.13938.pdf
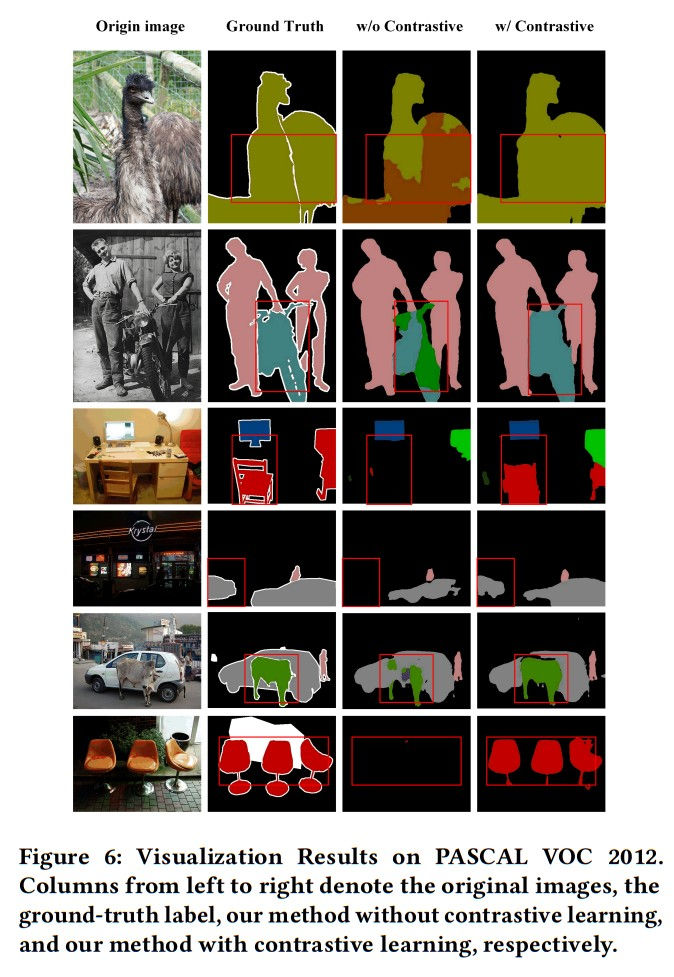
Semi-supervised semantic segmentation (SSS) is an important task that utilizes both labeled and unlabeled data to reduce expenses on labeling training examples.
... propose a dual-level Siamese structure network (DSSN) for pixel-wise contrastive learning.
By aligning positive pairs with a pixel-wise contrastive loss using strong augmented views in both low-level image space and high-level feature space, the proposed DSSN is designed to maximize the utilization of available unlabeled data.
... introduce a novel class-aware pseudo-label selection strategy for weak-to-strong supervision, which addresses the limitations of most existing methods that do not perform selection or apply a predefined threshold for all classes.
... strategy selects the top high-confidence prediction of the weak view for each class to generate pseudo labels that supervise the strong augmented views ... capable of taking into account the class imbalance and improving ... long-tailed classes.
... method achieves state-of-the-art results ... outperforming other SSS algorithms by a significant margin.
Please like and share this post if you enjoyed it using the buttons at the bottom!
Stay up to date. Subscribe to my posts https://morrislee1234.wixsite.com/website/contact
Web site with my other posts by category https://morrislee1234.wixsite.com/website
Comments