Survey of real-time object detection networks including versatility, robustness, resources, energy
- morrislee
- Feb 15, 2023
- 1 min read
Survey of real-time object detection networks including versatility, robustness, resources, energy
A Comprehensive Study of Real-Time Object Detection Networks Across Multiple Domains: A Survey
arXiv paper abstract https://arxiv.org/abs/2208.10895
arXiv PDF paper https://arxiv.org/pdf/2208.10895.pdf
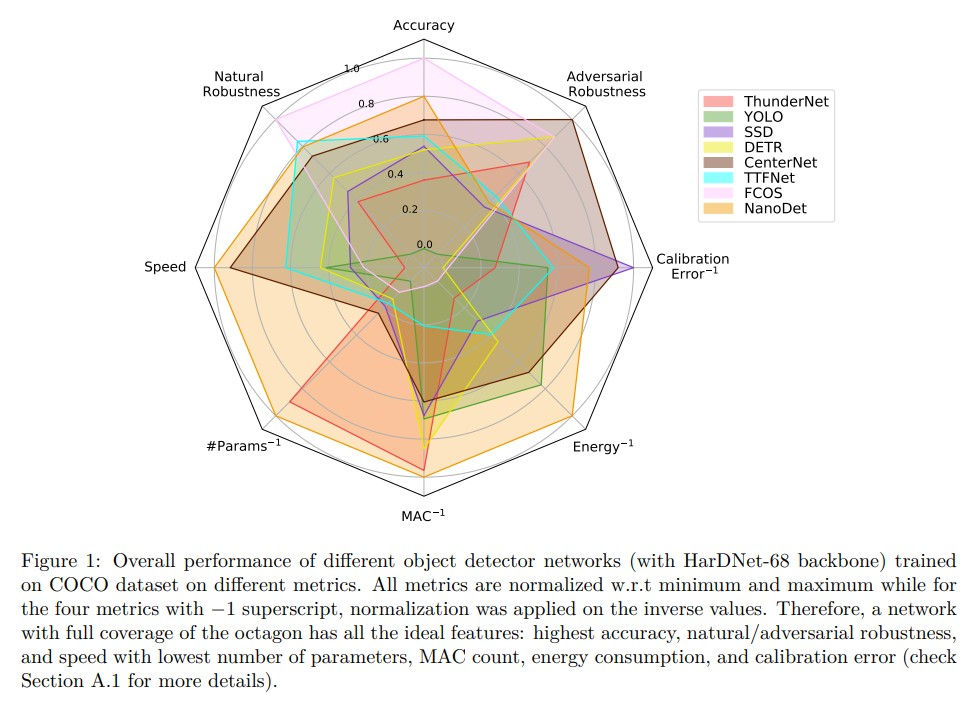
Deep neural network based object detectors are continuously evolving and are used in a multitude of applications, each having its own set of requirements.
... Real-time detectors, which are a necessity in high-impact real-world applications, are continuously proposed, but they overemphasize the improvements in accuracy and speed while other capabilities such as versatility, robustness, resource and energy efficiency are omitted.
... conduct a comprehensive study on multiple real-time detectors (anchor-, keypoint-, and transformer-based) on a wide range of datasets and report results on an extensive set of metrics.
... also study the impact of variables such as image size, anchor dimensions, confidence thresholds, and architecture layers on the overall performance.
... analyze the robustness ... against distribution shifts, natural corruptions, and adversarial attacks ... provide a calibration analysis to gauge the reliability of the predictions.
... To ... gauge the capability ... in critical real-time applications ... report the performance after deploying ... on edge devices ...
Please like and share this post if you enjoyed it using the buttons at the bottom!
Stay up to date. Subscribe to my posts https://morrislee1234.wixsite.com/website/contact
Web site with my other posts by category https://morrislee1234.wixsite.com/website
Comments